Tiny Mile: More Reliable Last Mile Delivery, at a Lower Cost
Optimizing Last Mile: How Tiny Mile, Manot, & Activeloop Increased Accuracy, Reduced ML Retraining Costs, & Streamlined Robot Delivery with Data-Centric AI
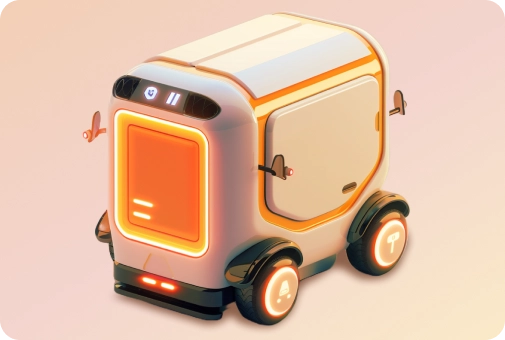
GPU Costs
About Last Mile Delivery
What is Last Mile Delivery?
Last mile delivery is the supply chain's final and most critical step, accounting for up to 53% of total shipping costs. As business-to-consumer deliveries increase, particularly from e-commerce companies, last-mile delivery has become a key focus in supply chain management. Despite high costs and outdated technology, innovative strategies and advanced technologies are being developed to address these issues and enhance customer experiences.
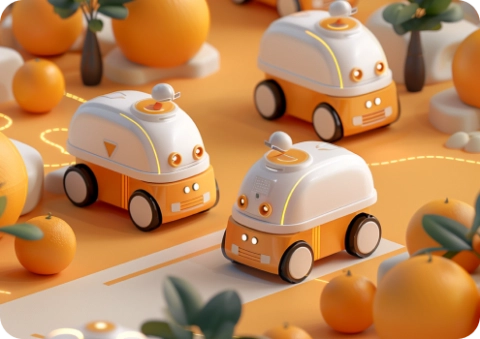
What is the Last Mile Problem?
Last-mile delivery poses several challenges, including high costs, real-time visibility, delayed or failed deliveries, outdated technology, decreased efficiency, and inefficient routing. The lack of quality last-mile delivery software makes it even more difficult to streamline the complexities of the last-mile delivery process. The future of last-mile delivery relies on cutting-edge solutions like autonomous delivery systems, representing a combination of autonomous or semi-autonomous delivery robots powered by machine learning.
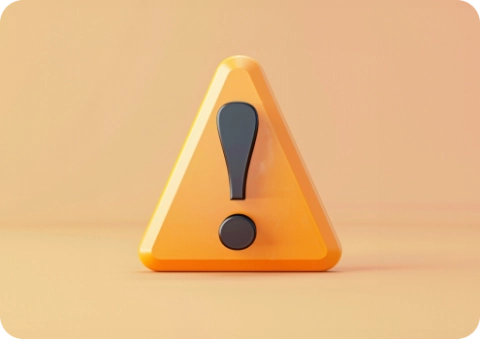
About the Company
Tiny Mile: Revolutionizing Last-Mile Delivery with AI-Powered Delivery Robots. Tiny Mile is a cutting-edge company specializing in last-mile delivery solutions using their distinctive small pink robots named "Geoffrey."
These AI-powered robots navigate sidewalks and streets in Miami and Charlotte, leveraging sophisticated computer vision systems to interpret their surroundings, avoid obstacles, and deliver packages directly to customers' doors.
To guarantee optimal performance and enhance the reliability of their robots, Tiny Mile partnered with Manot and Activeloop to monitor, observe, and explain their computer vision models in production and increase the efficiency of their data pipeline for model retraining, enabling Geoffrey to deliver packages more reliably.
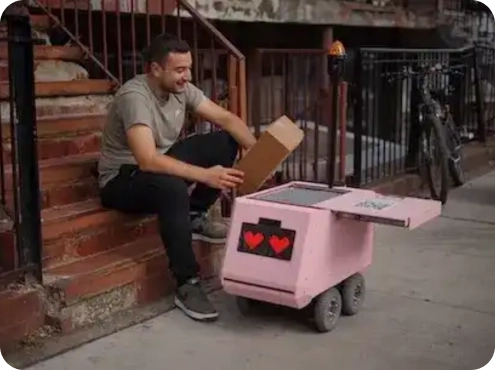
Meet the Interviewee
Ignacio Tartavull is the CEO of Tiny Mile, a last mile delivery robotics company dedicated to making robotics safe and accessible in modern living. With nearly 4 years at Tiny Mile, Ignacio leads a team passionate about creating respectful technology for cities and communities.
Prior to Tiny Mile, he worked on vehicle autonomy at Uber for almost 2 years and spent over 3 years in Computational Neuroscience at Princeton University's Seung lab. Ignacio's blend of technological expertise and ethical vision has shaped Tiny Mile into a recognized player in the industry.
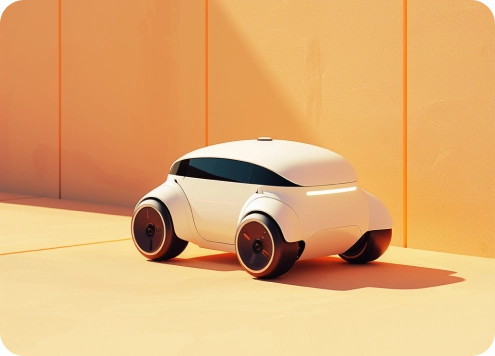
“Partnering with Manot and Activeloop has been a game-changer for us. By implementing their innovative solutions, we've been able to achieve improved efficiency and reduced costs, setting new standards in the last-mile delivery space. With their exceptional expertise in training performant computer vision models, we look forward to a continued fruitful partnership as we scale our ML efforts to revolutionize the future of delivery together.”
Ignacio Tartavull
Tiny Mile CEO & Founder
The Challenges
Overcoming Real-World Obstacles in Delivery Robot Performance. Deploying delivery robots with complex computer vision models that consistently operate in real-world environments presents significant challenges. Tiny Mile encountered difficulties in ensuring accurate object detection, efficient path planning, and dynamic obstacle avoidance due to.
- 1
Unexpected Scenarios
47% of the time, the in-house models faced data that wasn't accounted for during training. Real-world conditions are constantly changing, and training data cannot always capture every possible situation a robot may encounter.
- 2
Inaccurate Object Detection
Lighting conditions and object occlusion can affect the robot's ability to identify objects in its surroundings accurately.
- 3
Inefficient Data Pipeline
Tiny Mile needed to keep track of ever-evolving data and optimize the training pipeline to reduce costs, while scaling the training to millions of images.
- 4
Lengthy Feedback Loop
Identifying errors and fine-tuning the model for redeployment required a time-consuming process that slowed down the development and iteration cycles, hampering the company's time-to-market.
Solution
Enhancing Delivery Robot Performance with Manot and Activeloop. Tiny Mile partnered with Manot and Activeloop to establish a continuous feedback loop and implement an efficient data pipeline for model retraining.
Manot's system monitored the computer vision model's performance, identified outliers, and predicted unexpected scenarios where the robot could fail. When potential issues were detected, the corresponding images were uploaded to Activeloop's Deep Lake, triggering alert notifications for timely intervention and problem resolution. Manot helped enrich the Deep Lake dataset, filling gaps in the model's data to improve its accuracy in the real world.
With Deep Lake's visualization and query capabilities, the data was curated and efficiently streamed to machine learning models in real-time for retraining. Thanks to Deep Lake, the team could have a single view of the constantly updating dataset, keep track of changes with the version control, and efficiently train their models without worrying about idle GPU time or low GPU utilization.
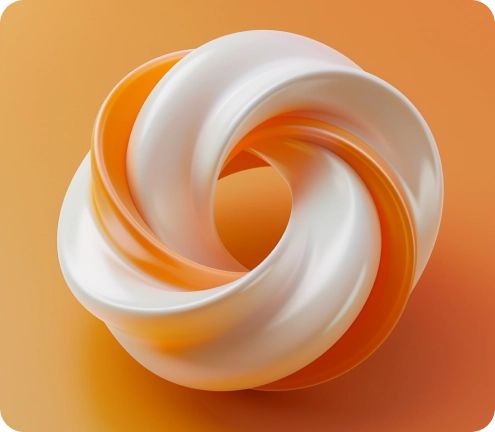
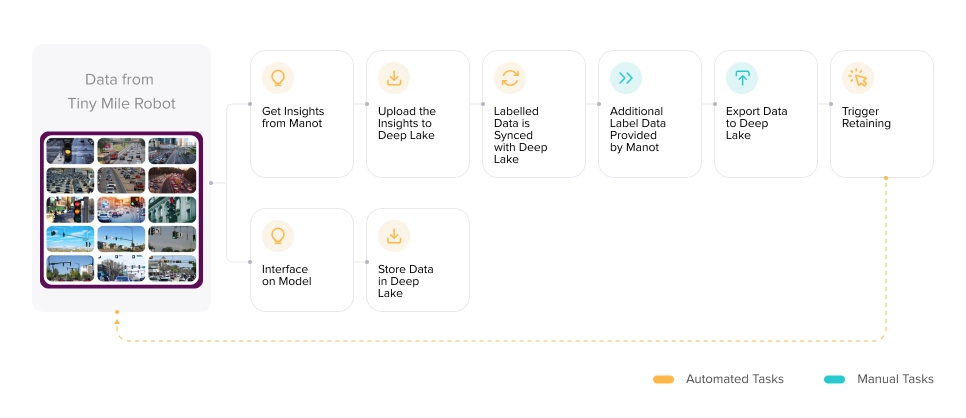
Results
10x improvement in time to production, and better model accuracy, at a lower cost. The partnership with Manot and Activeloop led to significant improvements in the performance and reliability of Tiny Mile's delivery robots:
Reduced Retraining Costs by 32%
The Automated Pipeline Decreased Model Retraining and Redeployment Costs.Increased Model Accuracy by 19.5%
Improved Accuracy in Predicting Potential False Positives and False Negatives, Ensuring Safer and More Reliable Robot Navigation.10x Improvement in Time to Production
The Continuous Feedback Loop Expedited Gathering Insights from Production to Development, Resulting in a More Efficient Delivery Process.
Future Plans: Reliable and Efficient Robots
Tiny Mile's collaboration with Manot and Activeloop has effectively addressed some of the key challenges associated with last mile delivery, such as high costs, or robot reliability in real world.
By implementing continuous monitoring, observability, and explainability systems, the performance of delivery robots has been significantly enhanced in real-world environments. The efficient and scalable data pipeline allowed to retrain the computer vision models used by Tiny Mile fast and at a lower cost, improving the time to market and cost-efficiency of the deep learning models powering the tiny robots.
In conclusion, this case study highlights the importance of embracing advanced technologies and innovative strategies to overcome the obstacles in last-mile delivery. By adopting this blueprint, other companies can revolutionize the last-mile delivery landscape, streamline their logistics processes, and ultimately enhance the overall customer experience. As technology continues to evolve, it is essential for businesses to adapt and leverage these advancements to stay competitive and meet the growing demands of the logistics industry.
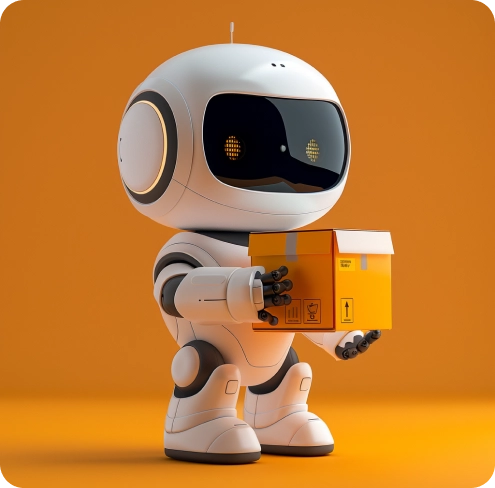
Improving Audio Machine Learning Infrastructure at Ubenwa
Learn how Ubenwa, a growing force in sound-based infant medical diagnostics, 2x efficiency & improved scalability with streamable, standardized Deep Lake datasets
Read more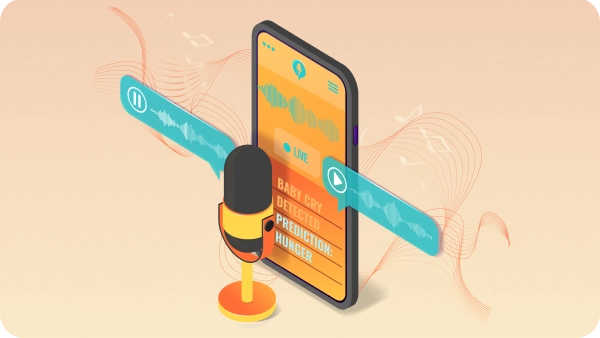
Increase in Lawyer Productivity with Hercules.ai by 18.5%
Discover how Ropers Majeski, a leading law firm, utilized Hercules.AI, powered by Activeloop's cutting-edge enterprise data solutions, to achieve remarkable productivity gains and cost efficiencies with LLMs
Read more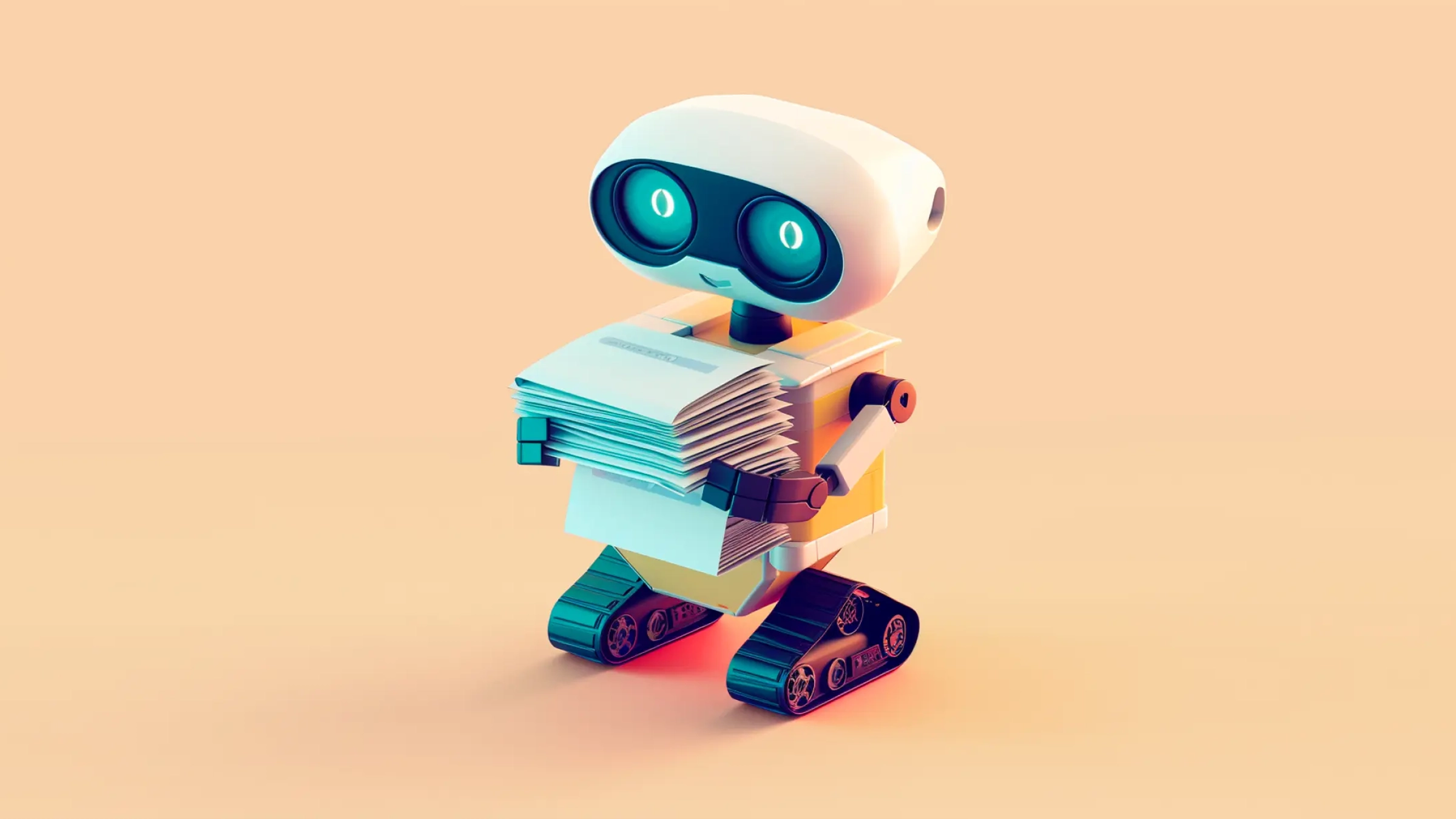