How Matterport Decreased Data Prep Times by 80% and Enabled Multimodal AI
Discover how Matterport leveraged Deep Lake to overcome data management challenges and expedite the training process of their machine learning models
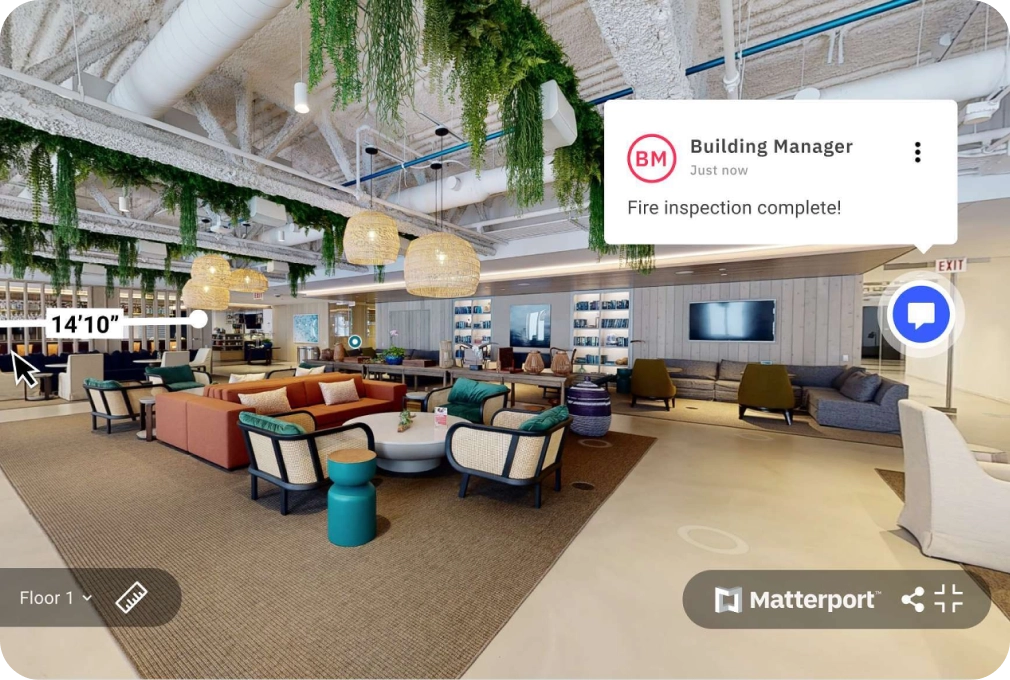
Data Prep
Matterport: Pioneers in 3D Digital Twin Technology
Matterport, a leader in 3D digital twins has digitized more than 35 billion square feet, making them one of the largest players in the domain. The company’s Vision & Learning team drives the company’s AI/ML capabilities. Alan Dolhasz manages the research activities of this team with a focus on computer vision and machine learning problems.
Furthermore, the team is responsible for rapidly assessing new research, converting promising results into fully fledged products that answer vital questions about the scanned spaces. They are at the core of Matterport's innovation, developing machine learning models on opt-in data to predict useful information about their spaces based on their extensive datasets1. Importantly, Matterport's highly selective approach to data utilization, aligned with customer privacy settings, ensures model accuracy and compliance amidst diverse data usage preferences.
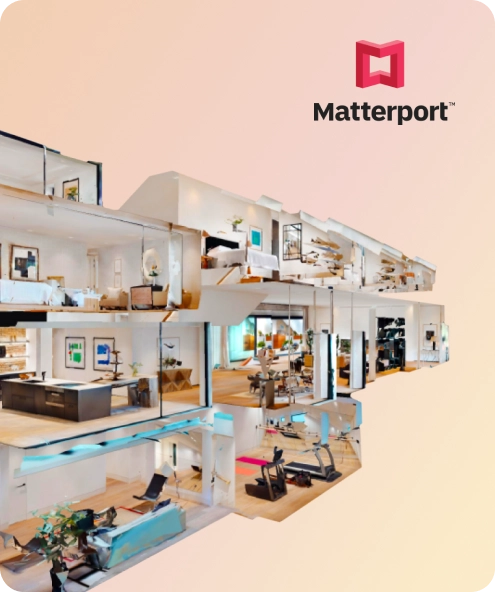
The Challenges
Before adopting Activeloop, Matterport faced challenges in managing their colossal datasets2. With over 7 million scanned spaces, the sheer size of the data posed significant logistical issues.
"Imagine you take a million Matterport spaces, each one might have a hundred photographs taken inside of it. You've got effectively two images that you need to store and maintain for every one of the 10 million items in the dataset. Very quickly, this becomes impossible to carry around"
Alan Dolhasz
Manager, Machine Learning Development at Matterport
- 1
Rapidly evolving vast data
The dynamic nature of Matterport's datasets3 introduced certain challenges. As Alan observed, "With every new engineer undertaking a project, there was an initial phase dedicated to transferring data, which, while necessary, involved a considerable amount of foundational work." This aspect of the process meant that a significant portion of time was invested in preliminary tasks such as data preparation and basic coding routines.
- 2
Lack of standardization
The absence of a unified standard in data management occasionally led to variations in how ML datasets4 were created, leading to a less streamlined approach across different projects. While this diversity in methods offered flexibility, it also underscored the potential for enhancing organizational coherence.
- 3
Experimentation and training models in the cloud
The process of setting up a new machine learning project was time-consuming since it involved downloading a large dataset5 from a cloud storage service like S3 and moving it back and forth. The transferring, storing, and tracking changes of these datasets were time-consuming and complex. As Alan highlighted, "Very quickly, as you scale up, this becomes super hard." This offered a valuable opportunity for streamlining processes within the dynamic environment at Matterport.
The Solution
With its capacity to handle multimodal data, Deep Lake significantly streamlined the data handling process for Matterport's machine learning projects.
Deep Lake just made it super easy for us to scale horizontally the different data modalities that we use.
Alan Dolhasz
Manager, Machine Learning Development at MatterportDeep Lake provided a uniform, efficient storage format for Matterport's datasets, allowing stakeholders across teams to store data in an ML-native format, and abstract away a lot of the boilerplate code required to set up a training pipeline for one project.
Deep Lake knocked out like 80 percent of the data random work associated... because once you've done it, that's it. Nobody else has to repeat that process unless you change the dataset.
Alan Dolhasz
Manager, Machine Learning Development at MatterportWith Deep Lake's streaming dataloader, Matterport was able to stream their data real-time to training frameworks, utilizing compute resources efficiently. With Deep Lake datasets acting as 'magic links' within the code, Matterport team was able to plug and play the dataset they wanted to rapidly iterate on choosing the best model architecture for the problem at hand.
With Deep Lake, it's literally changing one line and we can train on a completely different dataset. This is something that would take at least a day before
Alan Dolhasz
Manager, Machine Learning Development at Matterport
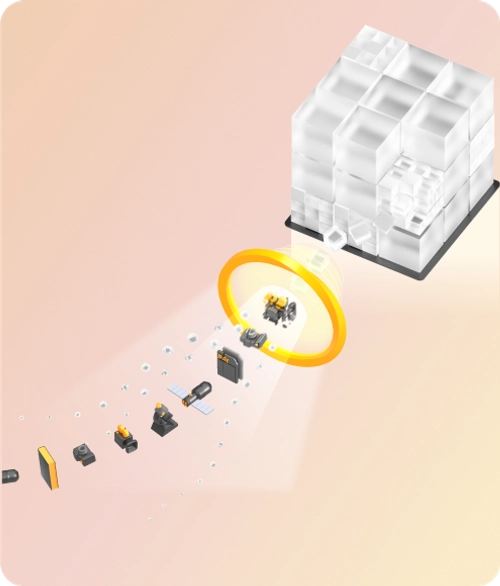
Data Visualization
Deep Lake's powerful UI for complex data visualization allowed the team to share datasets6 easily for QA among the team and with other teams who may not understand their work thoroughly.
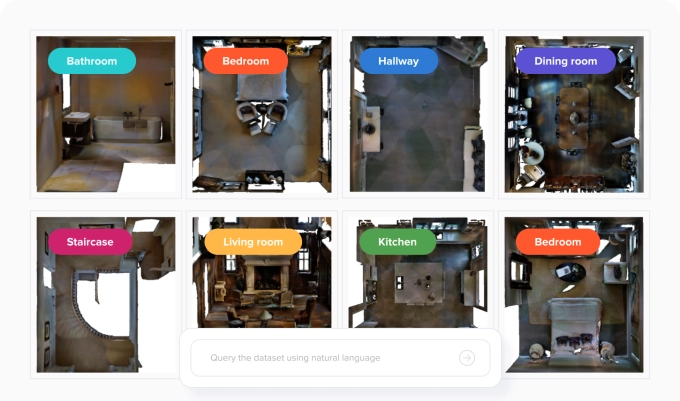
Results
Deep Lake significantly reduced the time and effort required to get from raw data7 to training. Implementing Deep Lake also led to substantial improvements in Matterport's operations, enabling the team to focus more on core tasks like iterating on model architecture and less on time-consuming data wrangling. It has freed up resources, and made managing complex, multimodal data easier.
It just abstracted so much of this work away so we could actually focus on the hard problems.

Alan Dolhasz
Manager, Machine Learning Development at MatterportIncreased Productivity
By standardizing the data handling process, Deep Lake allowed Matteport to allocate more of their time to business logic rather than infrastructure.-80% Less Time Spent
On Training Data PreparationFrom Hours to Seconds
Time to Train On a New Dataset“Deep Lake made working on more complex data no more complicated from a data management point of view. Whether I'm working on 10 million images with 10 different modalities or a thousand images with just one modality, it's all the same from the perspective of the user of the system.”
Alan Dolhasz
Manager, Machine Learning Development at Matterport
Future Plans
Combining generative AI and property insights, Matterport’s digital twin platform aims to reshape the real estate landscape, optimizing interior design, space utilization, energy efficiency, safety, and accessibility while transforming property marketing strategies.
The company is particularly focused on leveraging multimodal data to modify spaces based on user requests. As they dive deeper into this complex data, Deep Lake's ability to efficiently manage multimodal data will be instrumental in helping Matterport achieve its future objectives.
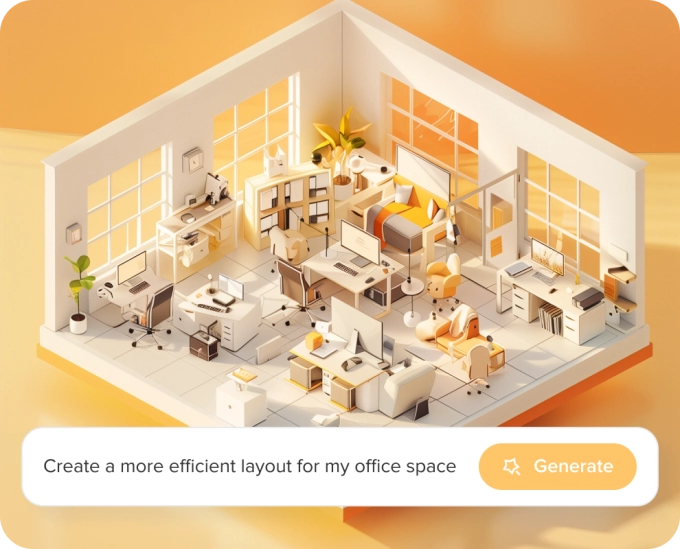
Disclaimer
1-7. Matterport is dedicated to using only authorized data to enhance and refine their services, with a strong commitment to respecting the privacy preferences of their diverse customer base. For further details, see Matterport's Terms of Use. https://matterport.com/terms-of-use
Increase in Lawyer Productivity with Hercules.ai by 18.5%
Discover how Ropers Majeski, a leading law firm, utilized Hercules.AI, powered by Activeloop's cutting-edge enterprise data solutions, to achieve remarkable productivity gains and cost efficiencies with LLMs
Read more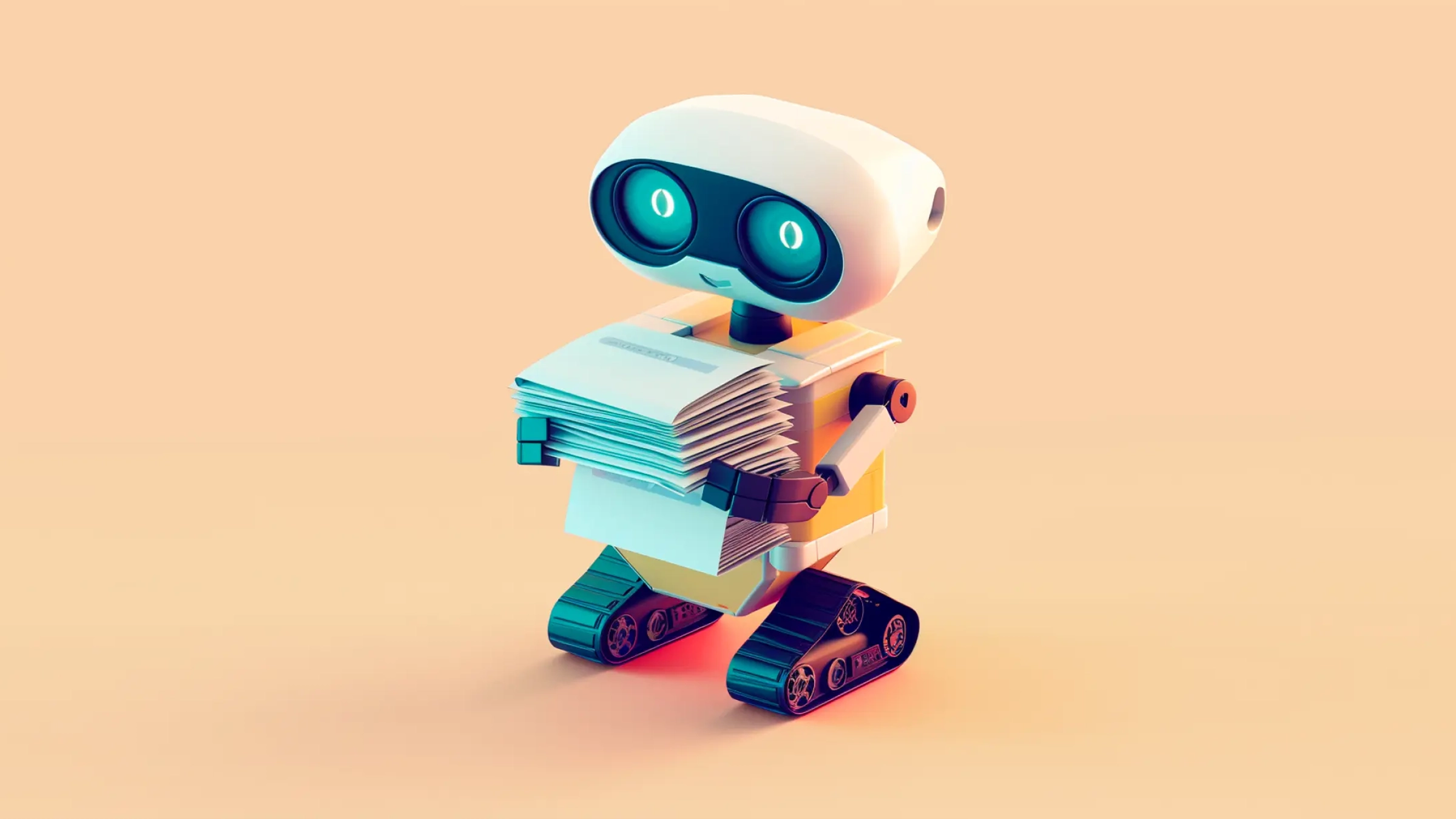
How Bayer Radiology Uses Database for AI to Disrupt Healthcare with GenAI
Learn how Bayer Radiology, a division of a pharmaceutical powerhouse, used a secure, efficient, & scalable database for AI to pioneer medical GenAI workflows
Read more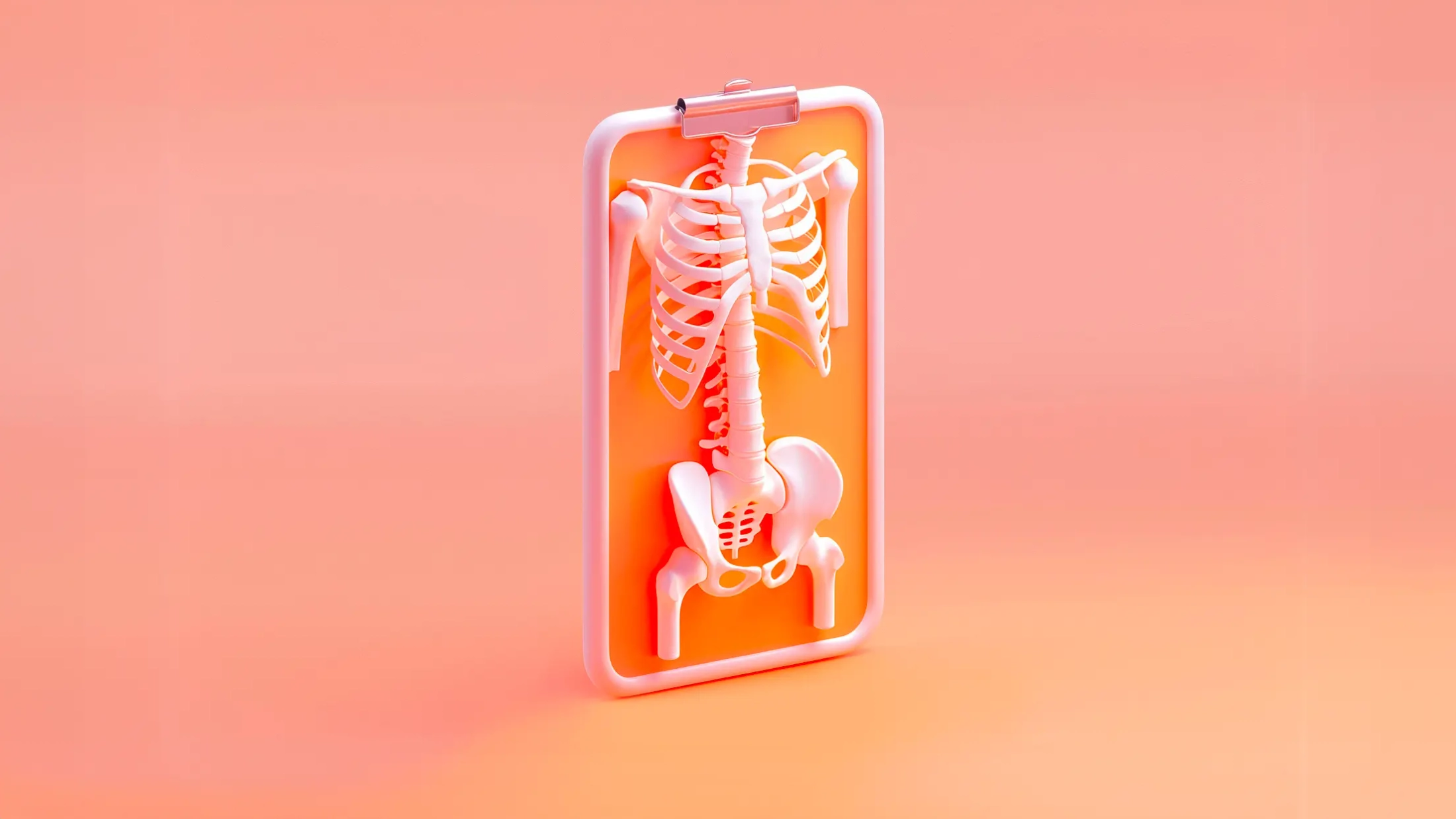