IntelinAir Faster AgriTech with Aerial Machine Learning Data Pipelines
Learn how IntelinAir, the leading crop intelligence company, transformed 1500 terabytes of aerial imagery into vital insights for farmers with scalable plug-and-play data pipelines with Activeloop and NVIDIA.
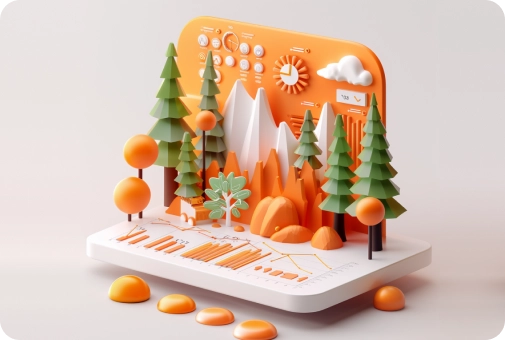
Inference Speed
Introducing IntelinAir
IntelinAir is a full-season and full-spectrum crop intelligence company focused on agriculture that delivers actionable intelligence to help farmers make data-driven decisions to improve operational efficiency, yields, and ultimately their profitability. IntelinAir, a member of NVIDIA Inception, combines the power of aerial imagery analytics through computer vision and deep learning methodologies, agronomic science, and user-friendly interface (mobile) technologies to deliver near real-time decision support to farmers.
Farmers simply cannot afford 1000 agronomists scouting their fields to detect pests, diseases, nutrition, and irrigation problems in their fields. That is where IntelinAir comes in.
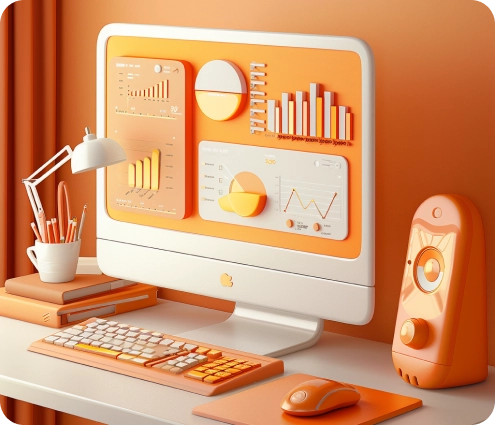
“Our goal is to organize and digitize the world’s crop data and performance - making it universally accessible and useful to boost yields, efficiency, farm sustainably, and feed humanity more effectively.”
Jennifer Hobbs
Director of Machine Learning, Intelinair
The Challenge
We are continuously collecting data. To rapidly deliver value to our customers, we need to develop, test, scale, and deploy our best-performing models to the cloud. Doing this consistently without reusable data pipelines is a Sisyphean task.
At IntelinAir, our data is big in several ways. First, we gather high-resolution imagery (10cm/pixel) which results in aerial images of >1GB for individual fields. Next, our unstructured data is multi-spectral and multi-sensor: in addition to RGB and NIR (infrared) imagery, we collect thermal, topography, soil composition, weather, and management (e.g. planter files and harvest maps) data.
Third, our data is temporal: we fly 13 flights across the season to understand how field health evolves and to capture how management decisions impact yield. This means that we have a lot of it: in 2020 alone, we will image millions of acres of farmland across hundreds of thousands of fields and capture over 1.5 petabytes (1,500,000 gigabytes) of raw data.
Having a stable, scalable pipeline in place was crucial to meet tight time limits from both our customers and Mother Nature

Jennifer Hobbs
Director of Machine Learning, Intelinair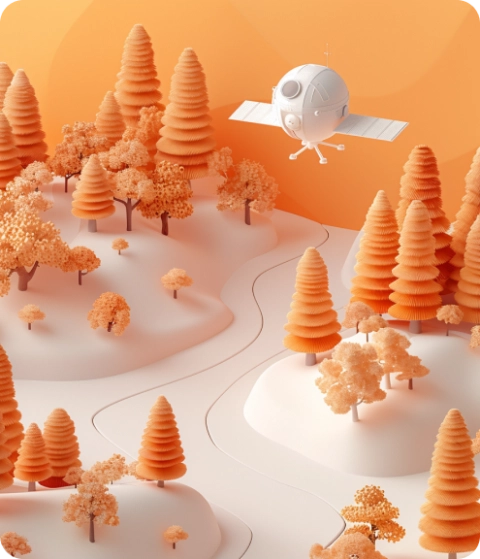
“1.5 PBs is a lot of data”, interjects Davit. “If one were to line up 1.5 petabyte's worth of 1 GB flash drives end to end, they would stretch across 138 football fields. 1.5 PBs equates to about 10 Billion, or 8.7% of all photos ever uploaded to Facebook. Intelinair is not alone in its search for ways to deal with big data more efficiently. In our experience, data scientists spend more than half of their time cleaning up the mix of structured and unstructured data and preparing to input it into the machine learning / AI models rather than actual big data analysis. At Activeloop, we managed to solve it by creating a fast and simple framework for building and scaling the data pipeline for IntelinAir.”
Data scientists spend more than half of their time preparing data for ML, rather than on actual ML.

David Buniatyan
CEO & Co-Founder at Activeloop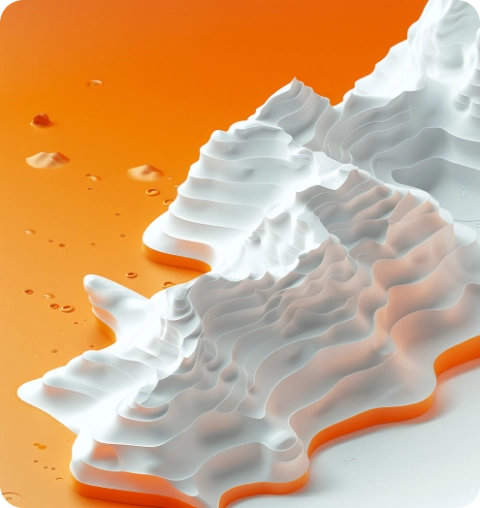
Faced with the challenge of managing vast datasets, the company focused on efficient data selection and pipeline management to minimize expenses. Instead of hiring more data scientists, Intelinair optimized their approach to data analysis by leveraging NVIDIA GPUs and Activeloop's database for AI, enhancing their analytics offerings while keeping costs down. They strategically balanced computing and network costs, choosing between local and cloud processing to maximize resource use. By experimenting with various data architectures and prioritizing reusable data pipelines, Intelinair achieved agility and efficiency in their operations, ensuring superior service delivery to their customers.
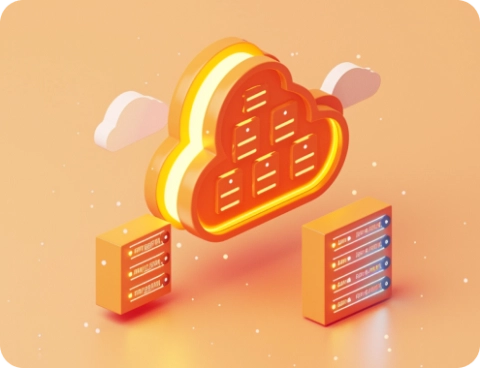
“To operate in an agile fashion, we want our data science team to focus on building high quality models instead of fighting with data pipelines, infrastructure, and deployment challenges.”
Jennifer Hobbs
Director of Machine Learning, Intelinair
Solution
Activeloop’s solution automatically ingests and preprocesses large volumes of data with scalable plug-and-play data pipelines. Intelinair can now easily build these pipelines, try them out locally, and then effortlessly scale to the cloud. We can directly stream generated datasets to deep learning frameworks for training machine learning models.
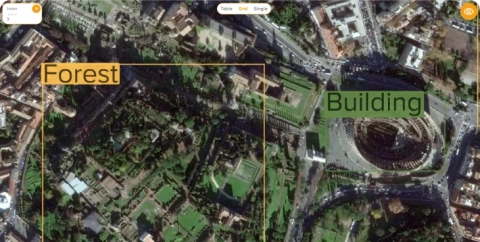
Leveraging Deep Lake, Intelinair created an automated platform for training classification models with a user-friendly interface, streamlining the process of training and comparing models like ResNet, DenseNet, and VGG by uploading flight-codes and labels. Activeloop handles auto-scaling and cluster management in the background, simplifying operations for Intelinair.
For advanced projects requiring object detection or segmentation, Intelinair sought precise control. Intelinair was able to store the data of different modalities, including annotations, flight data, and images in one unified way, which allowed for easy conversion of annotations into bounding boxes or segmentation masks, tailored to the model's requirements. This significantly cut down the time spent enhancing algorithm accuracy.
This is breakthrough stuff, letting us generate a dataset for our different tasks with just a few parameter changes, and get past the step of dataset creation, which is often the most time-consuming part of the model-building process. Intelinair can pull small datasets for debugging, as well as pull larger ones for experimentation and training, and do each one either locally or in the cloud as needed. With Deep Lake, Intelinair can pull only the data they need at a given point in time, saving on data access costs. With NVIDIA V100 GPUs, this also means they can crunch that data at the highest possible speeds, so training happens as quickly as possible.
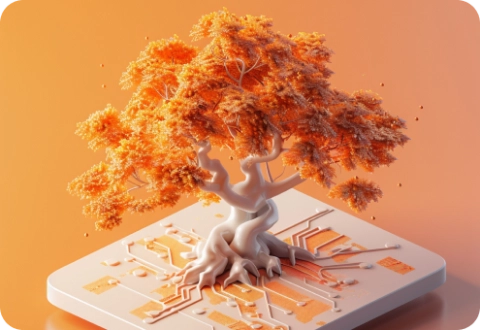
Results
The team at Activeloop works so fast. It's tremendous how quickly they are able to push out new features, address our concerns, and make improvements. We would discuss an idea one week, and it would be in production the next.

David Buniatyan
CEO & Co-Founder at Activeloop-50% lower Costs
For Compute and Storage Cost-30% Less Data Storage
Data Storage Required+12% Higher Accuracy
VS Baseline.95% at the End
“This whole project was all about empowering IntelinAir to do what they are best at - using their know-how in deep learning, computer vision, and agronomics. Together, we succeeded by contributing our expertise in processing data - intelligently and at scale.”
David Buniatyan
CEO & Co-Founder at Activeloop
Future Steps
The company aims to develop an extensive active learning platform that effectively "closes the feedback loop." By training models with existing annotations, deploying them for new data inference, correcting inaccuracies, and retraining with refined data, IntelinAir enhances the precision of its algorithms over time, especially with complex examples.
In this endeavor, IntelinAir is excited to partner with Activeloop, a collaboration aimed at bolstering all its models with this innovative approach. Committed to leading in the fields of machine learning and artificial intelligence, IntelinAir recognizes the forward-thinking visions of Activeloop. Through our collaborative efforts, IntelinAir is poised to significantly boost the agility and efficiency of its clients, paving the way for the future of agricultural innovation.
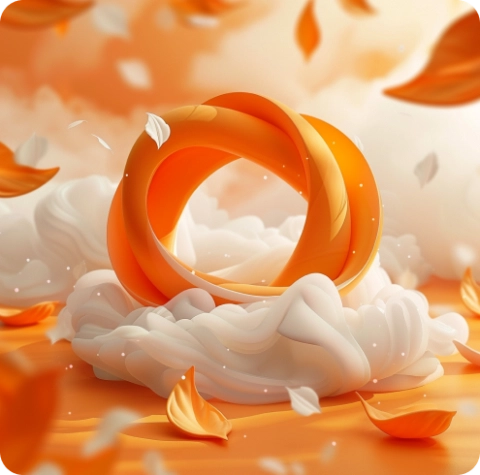
Improving Audio Machine Learning Infrastructure at Ubenwa
Learn how Ubenwa, a growing force in sound-based infant medical diagnostics, 2x efficiency & improved scalability with streamable, standardized Deep Lake datasets
Read more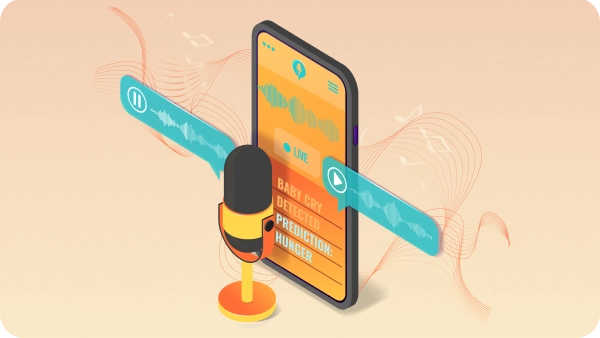
Tiny Mile: More Reliable Last Mile Delivery, at a Lower Cost
Optimizing Last Mile: How Tiny Mile, Manot, & Activeloop Increased Accuracy, Reduced ML Retraining Costs, & Streamlined Robot Delivery with Data-Centric AI
Read more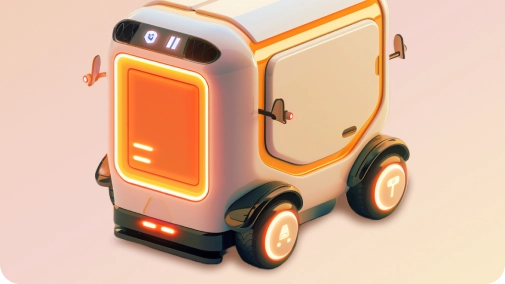