How Flagship Pioneering Makes Big Leaps in Biotech with Retrieval Augmented Generation
Learn how Flagship Pioneering & Activeloop are advancing retrieval augmented generation in biotech with a novel retrieval system for multi-modal biomedical data
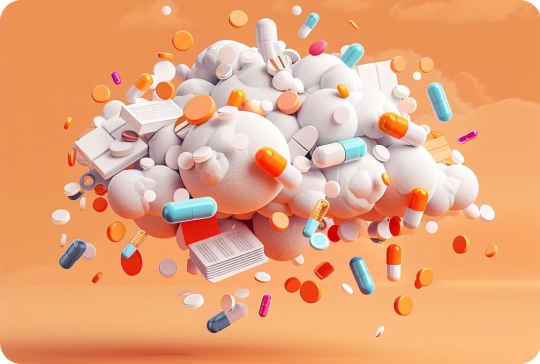
in retrieval accuracy
Introduction
Flagship Pioneering is a biotechnology company that invents platforms and builds companies focused on making bigger leaps in human health and sustainability. The company’s work ranges from applications in human health, such as designing new therapeutic modalities or early cancer detection, to tackling challenges in climate and sustainability, such as finding more resilient forms of agriculture.
Flagship’s innovations emerge from a systematic process of building and testing novel scientific and technological hypotheses. AI tools such as large language models have the potential to greatly augment such efforts, and thus, the ability to efficiently store and search across vast amounts of heterogeneous scientific data, especially in response to more broad and open-ended queries, is critical.
Flagship, and its Pioneering Intelligence (PI) initiative, as well as Activeloop embarked on a collaboration to develop novel tools to efficiently return highly accurate and useful scientific data in response to diverse scientific queries.
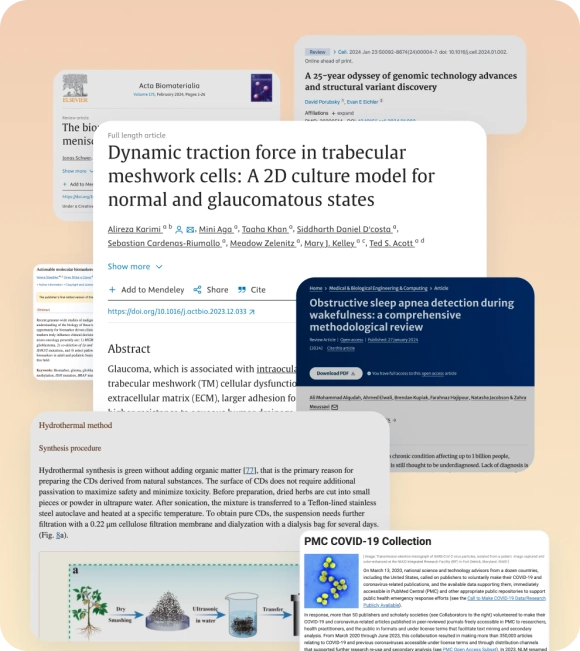
The Challenge
Retrieval augmented generation (RAG) is a powerful method for grounding large language models (LLMs) on specific evidence and reducing the likelihood of hallucinations. PI has been building various LLM-powered systems that leverage RAG to augment its scientific exploration process.
The efficacy of RAG is conditioned on the ability to provide LLMs with accurate and useful information. Existing RAG solutions fail when confronted with the massive scale and heterogeneity of biological data available and the extreme variety of potential biological queries. For example, there are over 30 million biomedical abstracts on PubMed alone — it is critical to implement an efficient storage and retrieval system for successful RAG applications.
Queries posed by Flagship during its exploration process often describe scientific ideas and concepts. Early experiments demonstrated that traditional search or keyword-based methods, despite performing well for queries about specific facts, struggled with queries that were more conceptual or open-ended.
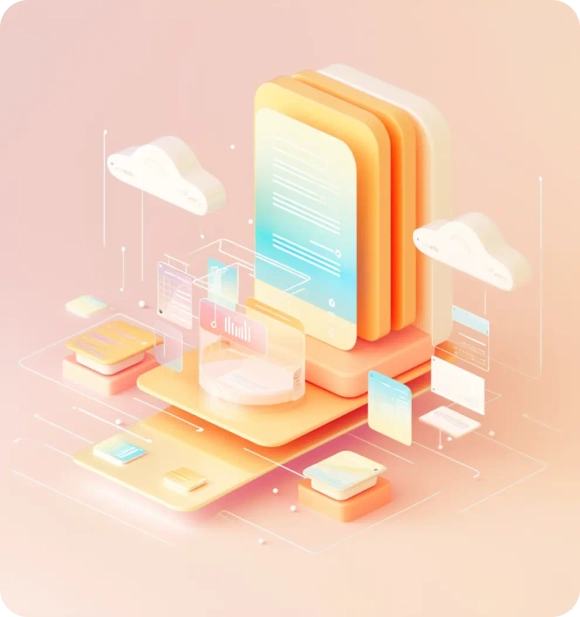
Activeloop’s Collaboration with Flagship’s Pioneering Intelligence
Together, Pioneering Intelligence and Activeloop formed a research partnership to address these needs. PI developed systems to generate and evaluate query-retrieval pairs at scale across a diverse range of biological topics. These queries were designed to reflect more “realistic” questions that Flagship might pose during scientific exploration.
Deep Lake and Deep Memory
Activeloop leveraged Deep Lake with its Deep Memory capability. Deep Lake is the database for AI that serves as a multi-modal vector store, while Deep Memory is an industry-pioneering feature of Deep Lake that increases retrieval accuracy without impacting search time with a learnable index from labeled queries tailored to a particular RAG application.
Flagship trained a custom Deep Memory model to make knowledge retrieval more accurate with their data.
Deep Lake and Deep Memory implementation are both remarkably easy. It's under ten lines of code to implement and get running
Ian Trase
Senior ML Research Engineer
Results
The trained system showed significantly increased accuracy over conventional methods. Additionally, Activeloop provided an easily implementable system for PI that reduced the time and cost of execution and enabled fast access to vast amounts of data.
"Comparing the Deep Memory-based model to baselines like Vector Search or Lexical Search, there was an average 18% improvement in the accuracy of the retrieved data sets. The gap was even more significant compared to the default search tools available on public databases. Additionally, Flagship was able to integrate the Deep Memory model into Flagship’s internal LLM application, providing a quick path to reach all users."
Mark Kim
Principal
18% Higher Accuracy
Compared to Baseline Vector or Lexical SearchUnder 10 Lines of Code
To IntegrateTens of Millions PDFs for RAG
Powerful Question Answering & Enterprise Search
Future Plans
Both Flagship and Activeloop are excited about the potential of expanding the system to handle more biological data types. Unexpected biological insights can emerge from “connecting the dots” between different data types such as text, tabular data, images, or graphs. However, there is currently no good way for biologists to semantically query and cross-reference multiple biological data types without significant manual coordination. Creating a system that automatically does that for these data types would drastically expand the scope of biomedical insights that could be built from available data.
The project’s success highlights the transformative potential of applying cutting-edge AI technology to enable fundamental capabilities. Despite storage and retrieval being relatively “basic” capabilities, the collaboration between Flagship and Activeloop has demonstrated the value of innovation in this space. By taking an AI-native approach to data storage and computing, new scale and scope for downstream applications become possible. As these capabilities expand to accommodate retrieval of more diverse, multi-modal data types, we anticipate even greater acceleration and augmentation of downstream applications.
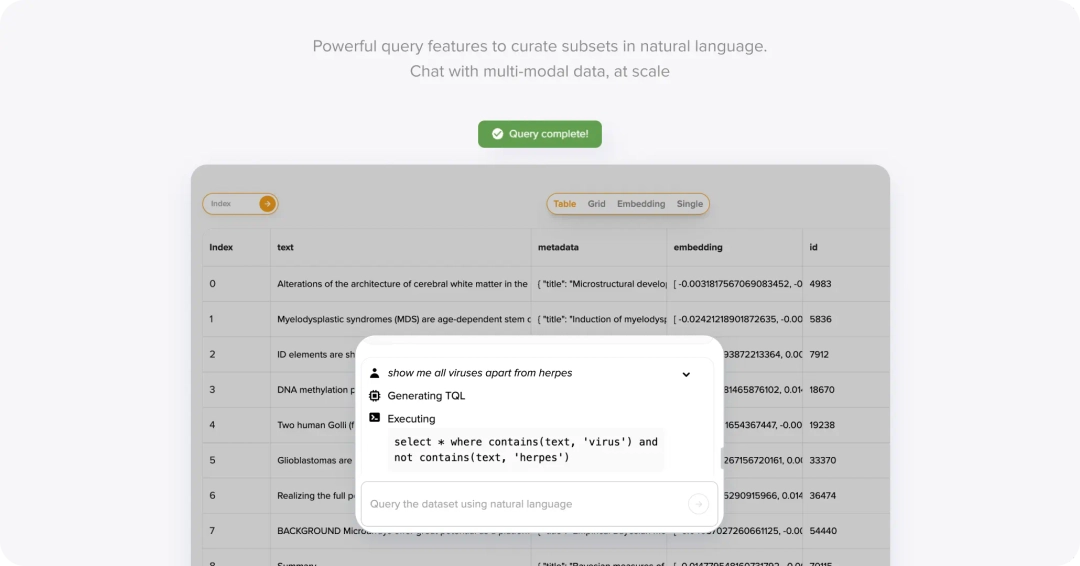
Powered by the Intel RISE Program
Activeloop and PI collaborated on this project under the Intel RISE program. This Intel endeavor enables industry leaders like Flagship Pioneering to harness cutting-edge technology to solve complex challenges in highly regulated industries – in this case, leveraging Intel CPUs for rapid vector store inference, allowing real-time filtering and retrieval for vast numbers of documents.
"One of the key things that enabled us to partner with Intel, the Disruptor Initiative, and the RISE program is to focus on key challenges for the next decade, including in biotech, pharma, healthcare, to solve them using AI."
Davit Buniatyan
CEO & Founder, Activeloop
"We were embarking on an ambitious research project to deliver a product that would be useful to scientists across the Flagship ecosystem. As with all research projects, a certain amount of iteration and exploration is needed at the beginning to decide whether we are tackling the right problem in the proper manner. Working under the Intel RISE program allowed us to explore."
Mark Kim
Principal
"In science, sometimes you have to rethink the basics to make progress. Flagship’s work with Activeloop has been all about that—getting back to the core of how we store and retrieve data for AI to speed up how we solve really tough scientific problems."
Mark Kim
Principal
Intel Disclaimers
Performance results are based on testing as of dates shown in configurations and may not reflect all publicly available updates. See backup for configuration details. No product or component can be absolutely secure.
Your costs and results may vary. For workloads and configurations, visit 4th Gen Xeon® Scalable processors at www.intel.com/processorclaims.
Results may vary.
Intel technologies may require enabled hardware, software or service activation.
Intel does not control or audit third-party data. You should consult other sources to evaluate accuracy.
Intel® technologies may require enabled hardware, software, or service activation.
© Intel Corporation. Intel, the Intel logo, and other Intel marks are trademarks of Intel Corporation or its subsidiaries. Other names and brands may be claimed as the property of others.
Increase in Lawyer Productivity with Hercules.ai by 18.5%
Discover how Ropers Majeski, a leading law firm, utilized Hercules.AI, powered by Activeloop's cutting-edge enterprise data solutions, to achieve remarkable productivity gains and cost efficiencies with LLMs
Read more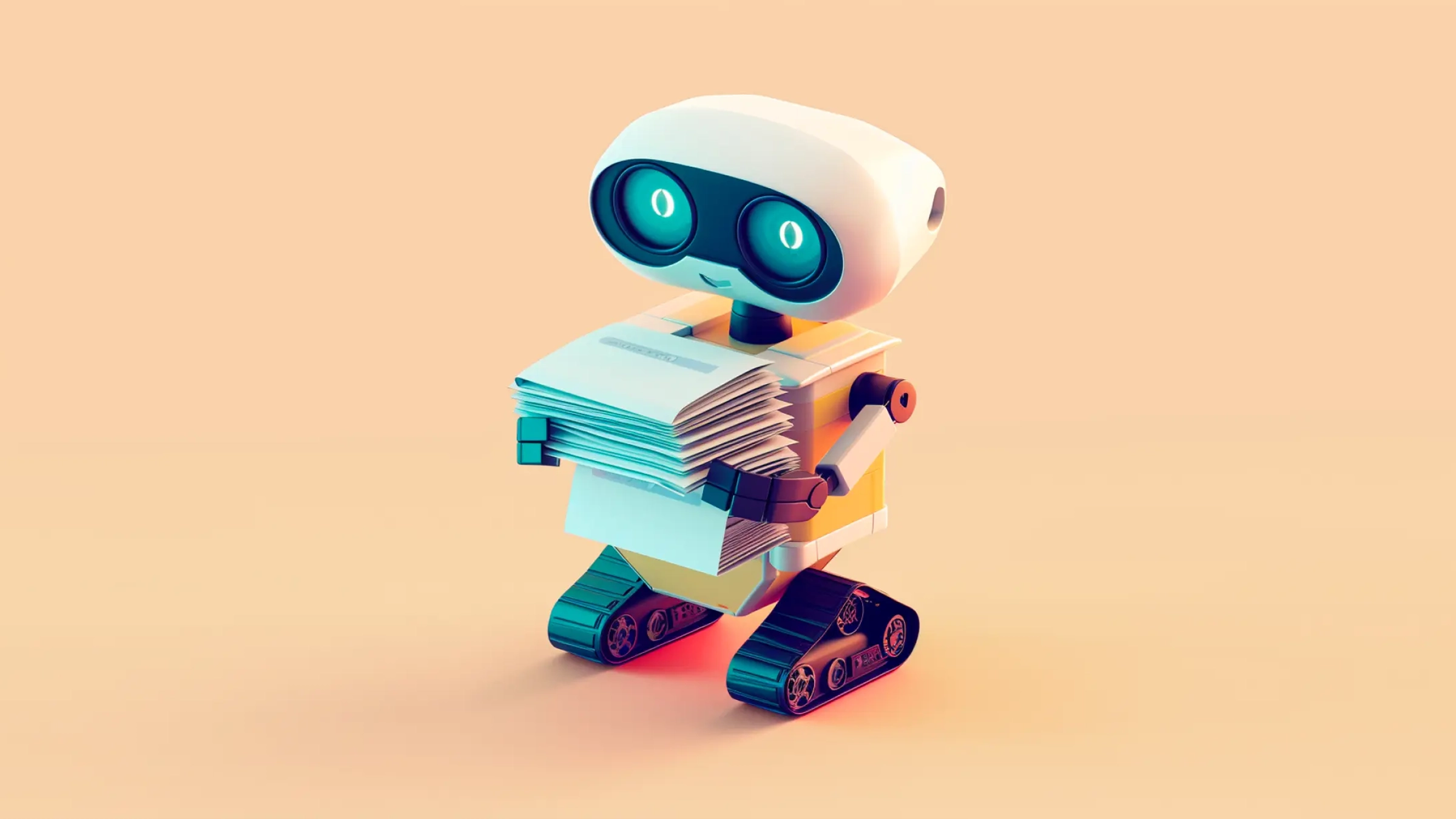
How Bayer Radiology Uses Database for AI to Disrupt Healthcare with GenAI
Learn how Bayer Radiology, a division of a pharmaceutical powerhouse, used a secure, efficient, & scalable database for AI to pioneer medical GenAI workflows
Read more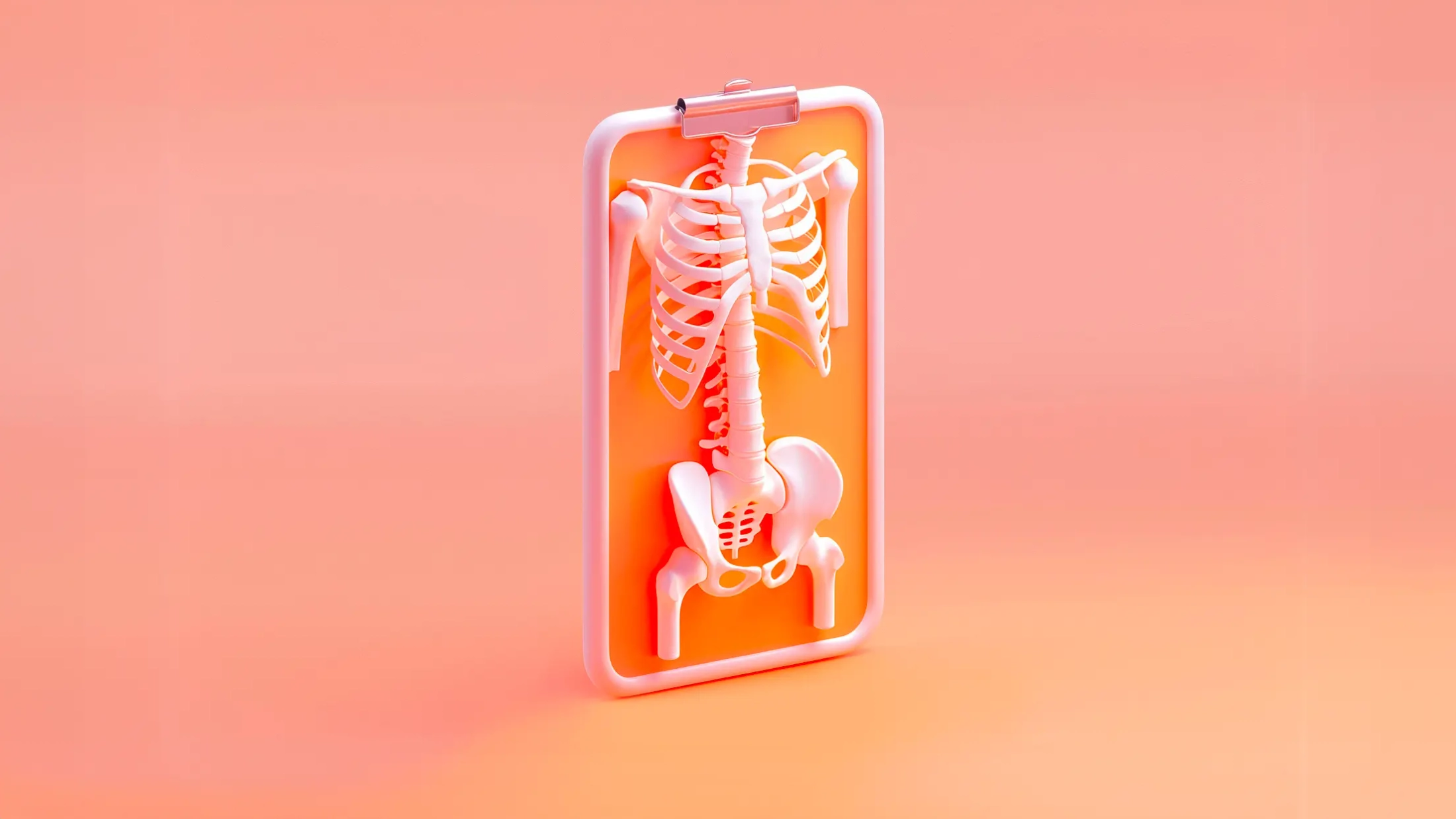