Manage Multimedia Datasets for Better ML Models
Achieve better image & video enhancement, face detection, sports analytics systems, AR/VR projects, & more
Used by
Image Enhancement, Image Restoration, Video Upscaling, Augmented Reality, Video Analytics, & Sports Analytics
Make more out of your content - either via enhancing & restoring it, or through extracting better insights. Database for AI allows using image, image sequence, video or audio datasets to create better & more engaging user experiences - from immersive AR to ball tracking
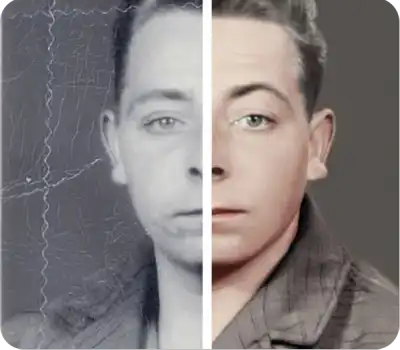
Image Enhancement
Make your users' images pop by building models to enhance the lighting, sharpness, or contrast of the images
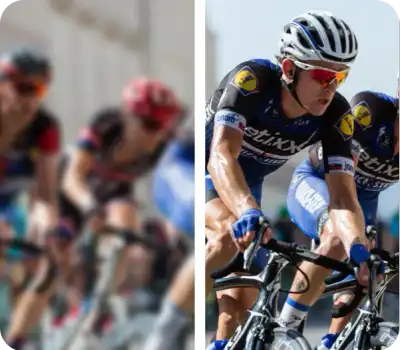
Video Enhancement
Build solutions to deinterlace, denoise, or upscale videos, & restore old media without adding visual artifacts
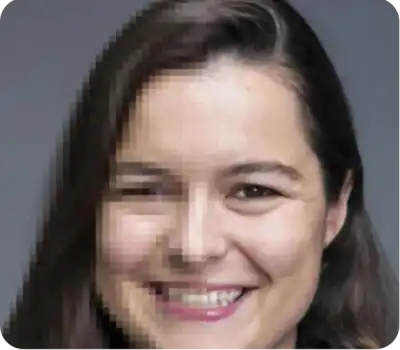
Image Restoration
Improve the quality of degraded (old, blurry, or noisy) images, reconstruct lost or decayed parts of images (image in-painting), or upscale images
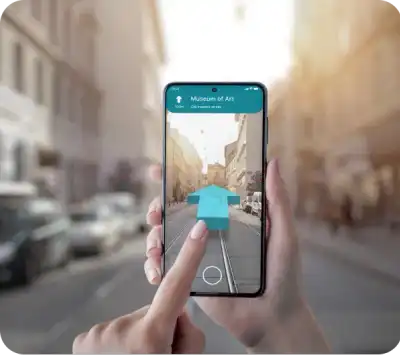
Augmented Reality
Deploy ML models to solve tracking, object or scene recognition, localization, & scene model construction tasks in e-commerce (AR for clothes or furniture try-on), entertainment (virtual cosmetics, face filters & digital avatars), gaming, & beyond
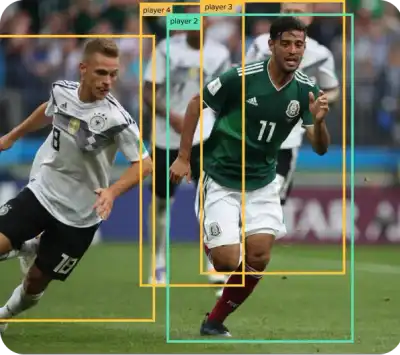
Live Sports Analytics
Analyze sports footage with computer vision models to understand the dynamically changing situation on the field, athlete performance, the sentiment of fans, or simply to deploy ball tracking systems
Machine Learning Datasets for Interactive & Informative Media
Don't have proprietary data? Get a head start by using one of the public machine learning datasets for image enhancement, image restoration, image upscaling, video enhancement, face detection, or live object detection for sports analytics.
"Facetuneā your ML workflow. Use data infrastructure for your multimedia use cases with Activeloop
With the rise of AI in multimedia, companies craft more pleasing experiences for their users, sports fans, or consumers - at scale.
This can be achieved in three main ways. Firstly, data scientists can help improve and restore deteriorated or corrupted content. They tackle tasks such as image restoration (image upscaling, image reconstruction, in-painting), image enhancement, and video enhancement (e.g. video denoising, video enhancement, or video stabilization). Secondly, ML teams may focus on enabling users to generate more content or interact with it. Common tasks include facial detection, facial landmark estimation, human pose estimation, or object segmentation. These can provide social media users with exciting new filters, or help them try on virtual clothes or furniture. Thirdly, ML engineers may focus on extracting insights from live video feeds - to analyze sports footage, track athlete performance or objects like balls in real-time, and gauge viewer sentiment and engagement.
Activeloop helps machine learning teams applying deep learning in multimedia space ship their AI products fast. ML engineers can ingest raw multimodal datasets (image, image sequences, video or audio) with the metadata to materialize datasets that can be streamed while training in ML frameworks with one line of code. Also, ML teams can collaborate on curating their datasets by rapidly querying subsets of even petabyte-scale data in seconds.